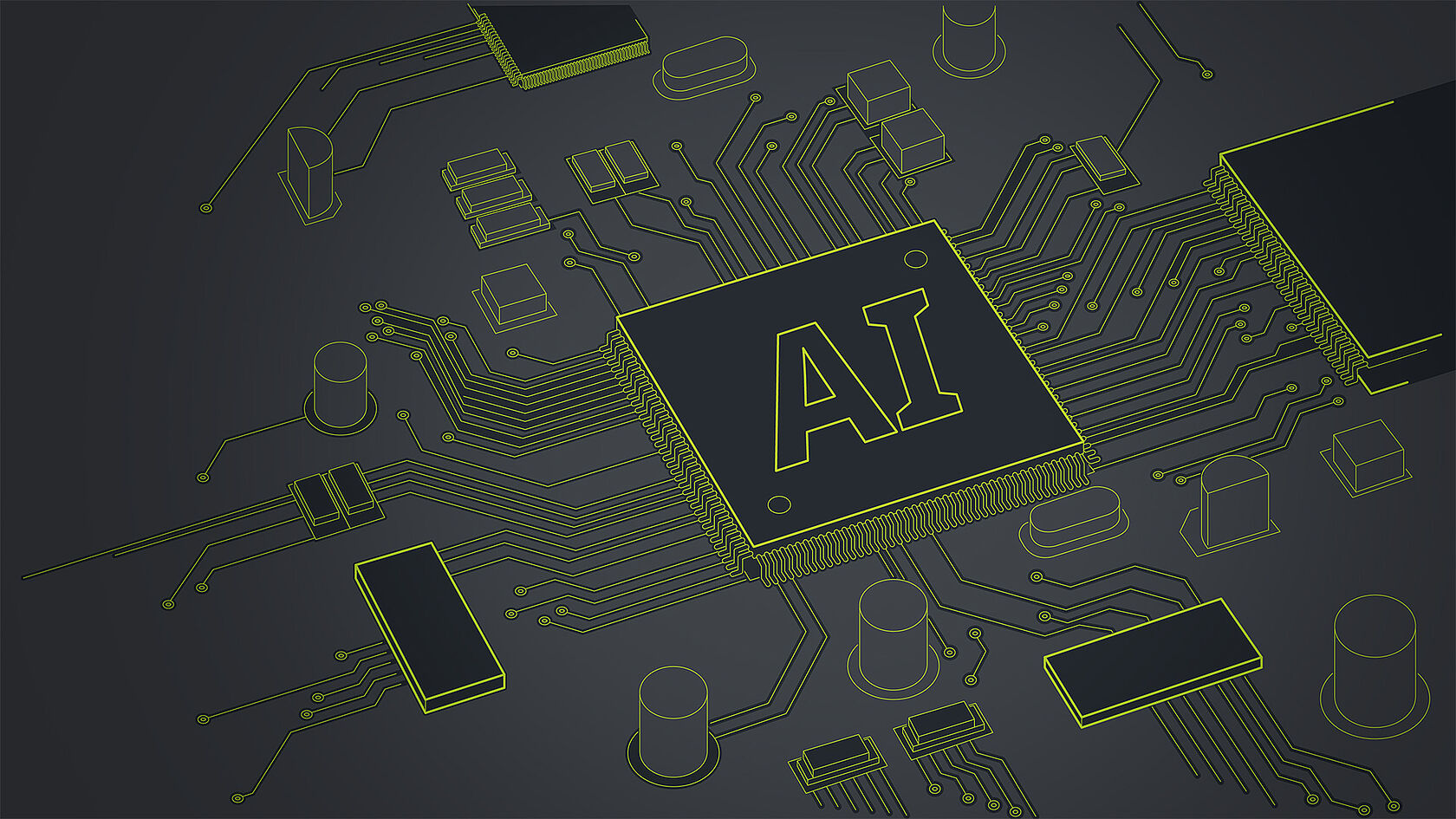
Industrial AI Use artificial intelligence to optimize and automate business processes
Gain competitive advantage with industrial intelligence.
Challenges in using industrial AI
The use of artificial intelligence (AI) offers enormous potential for efficiency and productivity, for example in the planning and visualization of work processes or in the maintenance of systems. Because this creates decisive competitive advantages, the pressure on companies to act is constantly increasing. At the same time, the widespread use of AI applications in industry is not yet a reality. This is because process owners are not fully willing to trust the forecasts or automated decisions of a supposed AI black box and are reluctant to integrate corresponding applications.
Easy AI solutions for all companies
Many companies do not have the time or resources to build up comprehensive knowledge about artificial intelligence on their own. Therefore, AI solutions are required that are easy to understand, explain and intuitive to use. These solutions should take into account the different levels of knowledge of different user groups.
-
Method combination
Only in combination with conventional optimization methods does AI fully play out its advantages.
-
Data robustness
Business processes must continue reliably even with incomplete data. This is where AI comes in, providing the best decision support even under difficult conditions.
-
Process integration
AI is not a stand-alone application. It must be securely integrated into existing processes and interact with other services via interfaces and protocols.
Use of artificial intelligence in industry
Companies use Industrial Intelligence to gain significant advantages over their competitors. By cleverly combining various AI methods, they can
- continuously save time and costs,
- optimize and automate routine processes,
- increase productivity and efficiency,
- improve sustainability and
- make faster and better decisions.
See how our customers have used AI to improve their businesses
Productivity gains
Cost savings
Emission reduction
References
Industrial AI product solutions are in productive use at many PSI customers across all industries.